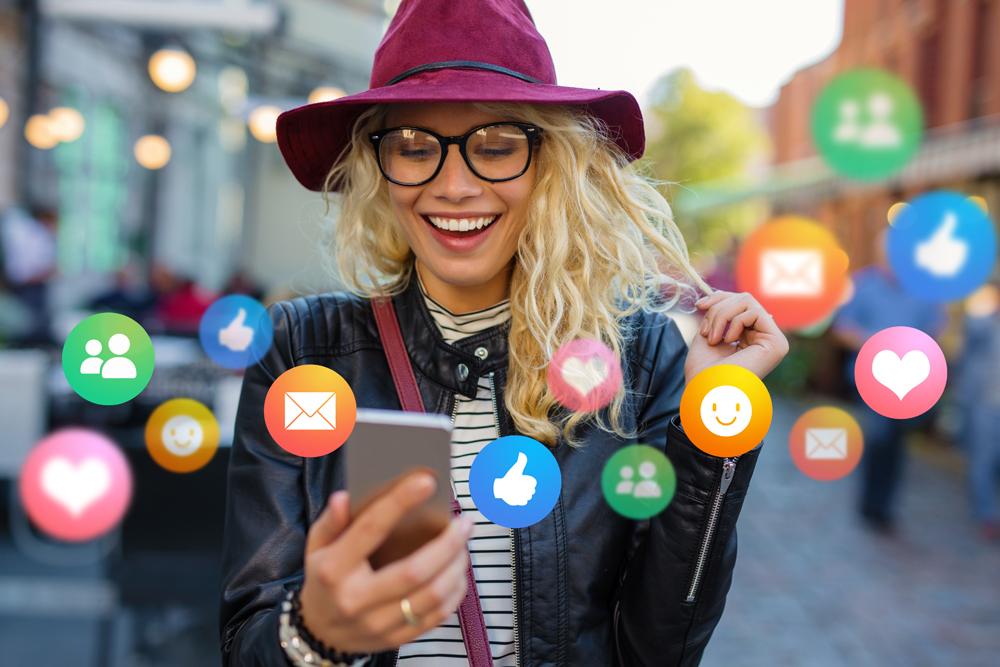
Predictive Analytics has become an indispensable tool for businesses seeking to forecast industry trends, understand customer behavior, and preempt potential risks. This advanced form of analytics uses historical data, statistical algorithms, and machine learning techniques to identify the likelihood of future outcomes. This blog explores how predictive analytics is shaping industry trends, especially loyalty industry, influencing customer interactions, and mitigating risks across various sectors.
Enhanced Customer Segmentation
Predictive analytics is revolutionizing the loyalty industry by enabling businesses to personalize customer interactions, enhance engagement, and ultimately, increase retention rates. Traditional customer segmentation often relies on broad categories such as age, gender, or purchase history. Predictive analytics goes several steps further by using advanced algorithms to analyze more nuanced data, including browsing habits, social media interactions, and past communication responses. This allows businesses to create highly specific segments and tailor their loyalty programs to address the distinct preferences and behaviors of different customer groups, making the programs more relevant and engaging.
Forecasting Industry Trends
Predictive analytics has the power to transform a broad spectrum of industries by forecasting market trends with a high degree of accuracy. For example, in the retail industry, predictive models can analyze seasonal buying patterns and consumer behavior to predict future sales trends. This allows retailers to optimize their stock levels, tailor marketing campaigns, and adjust pricing strategies in real-time, staying one step ahead of consumer demand and market dynamics.
Personalized Rewards and Offers
One of the most direct applications of predictive analytics in the loyalty industry is the personalization of rewards and offers. By predicting what specific customers are likely to purchase in the future, companies can customize their offers to match individual tastes and preferences. This increases the perceived value of the loyalty program and enhances the likelihood of repeated engagements and purchases. For example, a predictive model might identify that a customer frequently purchases eco-friendly products and could suggest offering special points or discounts on similar items.
In e-commerce, predictive analytics enables businesses to create personalized shopping experiences by recommending products based on browsing and purchase history. This not only enhances the customer experience but also increases the likelihood of conversions.
Risk Management and Mitigation
Risk management is another area where predictive analytics can add significant value. By forecasting potential risks and their impacts, companies can devise strategies to mitigate them before they manifest. In the supply chain sector, predictive analytics can forecast potential disruptions caused by external factors such as economic downturns or natural disasters. This foresight allows companies to adjust their inventory and logistics strategies to ensure business continuity.
Healthcare providers use predictive analytics to improve patient outcomes by predicting risks of diseases or readmissions. This not only helps in providing better care but also reduces the costs associated with treatment and hospitalization.
Churn Prediction and Prevention
Customer churn represents a significant challenge in the loyalty industry. Predictive analytics can identify at-risk customers before they defect. By analyzing data points such as changes in buying frequency, decreases in average order value, and sentiment analysis from customer feedback, predictive models can flag customers who might be losing interest. Businesses can then proactively engage these customers with special offers or personalized communications to reinvigorate their interest and retain their loyalty.
Future Spending Predictions
By understanding a customer’s past purchasing patterns, predictive analytics can forecast future spending behaviors. This insight allows companies to predict lifetime value and potentially tailor the loyalty program to maximize the long-term profitability from high-value customers. Moreover, it can help in allocating marketing efforts more efficiently, focusing on nurturing potentially high-value customers.
Real-time Engagement Strategies
With the rise of mobile technology and integrated data systems, predictive analytics can also enable real-time engagement with customers. For instance, when a loyalty program member enters a store, predictive analytics can instantly analyze their purchase history and current promotions to push a personalized offer to their smartphone, enhancing the likelihood of a purchase during that visit.
Conclusion
Predictive analytics is reshaping how businesses forecast trends, understand customers, and manage risks. By converting raw data into actionable insights, companies are not only able to make smarter decisions but are also better positioned to anticipate and respond to changes in the marketplace. Incorporating predictive analytics into loyalty programs offers a competitive edge by enabling a more personalized, responsive, and effective approach to customer loyalty.
of our story
hr@annexcloud.com